Detecting Clouds: How Machine Learning Improves Field Health Maps
August 6, 2019
Has this ever happened to you? You pull up a field health image and find that your scouting map is almost completely red. You go out and scout the field, expecting devastation and to your surprise, everything is perfectly fine. This is a classic example of cloud blockage - clouds are quite literally getting in the way of field health images.
Unclouding the View
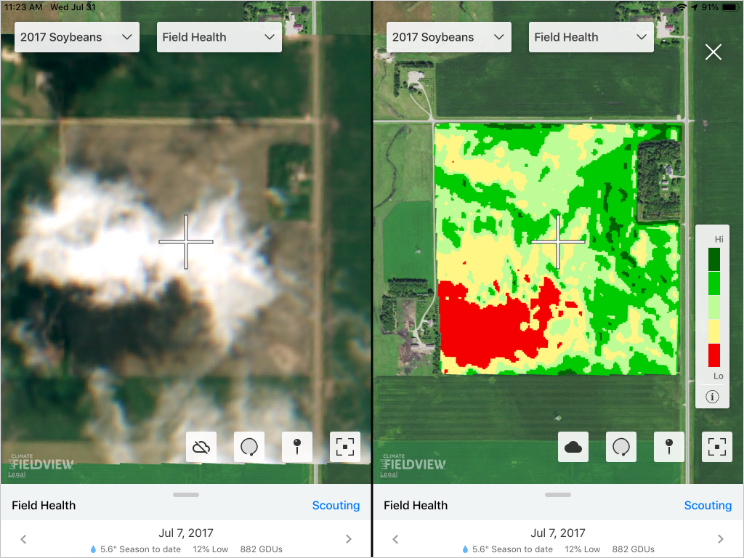
Above is an instance of cloud blockage. The image on the left is the real-life cloud image. The image on the right is the FieldView™ map prior to our current cloud detection technology. The large red spot is where the cloud is either casting a shadow or obscuring the view of the satellite taking the picture.
When clouds obstruct the view of satellites, the technology that analyzes images to produce scouting maps and other data interprets this as a field health problem. This results in misleading field imagery. As a data scientist, the last thing I ever want to do is provide inaccurate data. So my colleagues and I set out to solve this issue by creating better, more sophisticated technology.
"We developed a way for machine learning to detect and identify cloud blockage."
We developed a way for machine learning to detect and identify cloud blockage. First, we needed to help FieldView™ learn what clouds look like. Our field health imagery tool was built for analyzing crop imagery. So in a sense, we had to “teach” or “train” FieldView to identify a cloud. This process is commonly known as “machine learning.” But how do you teach technology?
As people, we look at a picture as one object. A computer, on the other hand, will process an image as a collection of pixels. When FieldView receives a field health image, it scans every single pixel in the picture and looks for specific colors and patterns. When it sees a white cloud or dark shadow, it highlights this as a potential field health issue, when in actuality, it may not be.
Our solution was to create what’s called a “classifier” to automatically separate the parts of the picture that show signs of cloud blockage. We taught the classifier to do this using hundreds of thousands of images of clouds of different shapes, sizes and transparency. These images were analyzed across different landscapes and times during the growing season, helping the classifier learn to identify them. This innovation enables FieldView to provide a valuable field health image even if part of the picture was compromised by clouds.